Discovering New Sounds: A Guide to Similar Music Finders
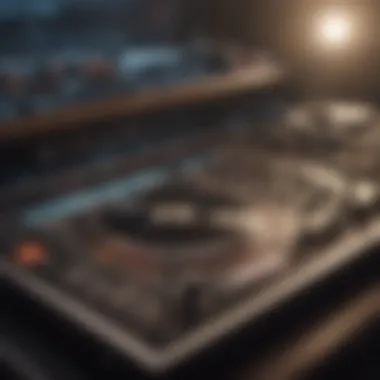
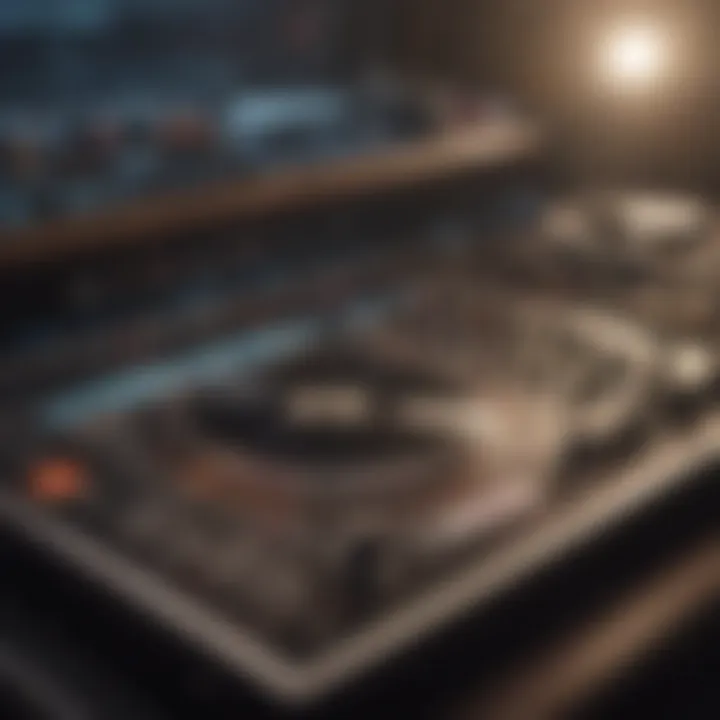
Intro
Navigating the ever-expanding realm of music requires tools that assist in music discovery. With so many genres, artists, and styles, identifying new sounds that match one’s preferences can be daunting. This article focuses on similar music finders, which are gaining traction in facilitating the exploration of new auditory landscapes. By suggesting tracks and artists that resonate with the user’s established tastes, these platforms simplify the journey of finding fresh music.
We will explore the technology at play, the efficacy of these finders, discuss their merits and shortcomings, and provide insight into enhancing music search through a better understanding of genre and mood variations. The ultimate aim is to equip music enthusiasts with knowledge that leads to diverse and enriching listening experiences.
Overview of Similar Music Finders
Music finders use directly applied algorithms to assess user preferences. By analyzing listening patterns and choices, these tools curate playlists or suggest songs that cater to the user's unique auditory taste.
The emergence of streaming services such as Pandora, Spotify, and Apple Music indicates a growing trend in music consumption that emphasizes personalized discoverability. Listeners are shifting from passive enjoyment—where they simply play popular tracks—to a more engaged method of exploration often guided by these finder tools.
“Similar music finders tap into the wealth of existing content, reducing the noise involved in discovering new favorites.”
Algorithms Behind the Finders
The sophisticated algorithms powering these music finders are generally categorized into two main types: content-based filtering and collaborative filtering. Each approach presents various methods for how tracks are matched:
- Content-based filtering: Focuses on the actual features of the songs. For instance, it might analyze tempo, key signature, and stylistic attributes to find matching tracks.
- Collaborative filtering: Rely on analyzing listening habits not just of the user but also the wider community. This makes use of trends among similar users to predict what might intrigue a listener based on popular shared choices.
These practices can markedly enhance users’ ability to uncover tracks that align with their tastes, enriching their musical journey.
Advantages of Similar Music Finders
- Expedited Discovery: Finders allow users to bypass saturated playlists by steering them to lesser-known tracks.
- Expanded Taste: Encouraging exploration helps users to step outside their comfort zone and engage with various genres and styles.
- Personalized Recommendations: Tailoring suggestions opens the way to creating a more vibrant music environment tailored to individual tastes.
However, it is crucial to note that users still need to engage actively, as technology does not wholly replace personal taste.
Limitations of Music Finders
Discrepancies do arise with these music finders.
- Algorithmic Bias: Systems may underestimate diversity, favoring popular choices unheard of previously instead of niche artists.
- Repetitive Suggestions: Users may find they receive similar recommendations frequently, which can hinder true discovery.
Awareness of these limitations can lead users to approach similar music finders critically, helping them maximize their potential benefits.
Epilogue
Similar music finders can immensely assist listeners searching for new sounds that resonate well with their favored vibes. Understanding these tools, their strengths, and challenges is essential for fans who wish to enhance their listening experiences. Through careful engagement with these platforms, users can cultivate a richer and more varied musical landscape.
Understanding Similar Music Finders
Understanding similar music finders provides an essential framework for those seeking new auditory experiences. The evolution of these tools reflects the dynamics of an ever-changing musical landscape. By utilizing technology, these platforms assist users in discovering songs and artists that resonate with their tastes. With the variety of genres available today, finding new music without a guided approach can be daunting.
Definition and Purpose
Similar music finders are digital tools designed to recommend music based on user preferences and listening habits. They use complex algorithms to analyze user data, enabling them to suggest tracks that align with a user's established tastes. The purpose of these tools goes beyond just finding new songs; they also aim to enhance the overall listening experience. Users can explore various genres, dive into related artists, and benefit from customized playlists. As such, these platforms form a crucial part of modern music consumption.
Importance in the Music Industry
The significance of similar music finders in the music industry cannot be overstated. They offer numerous benefits to listeners and creators alike. For consumers, these tools act as a means of exploring new sounds efficiently. Conventional radio or playlists can limit what listeners hear. Conversely, similar music finders expose users to a broader selection. This exposure is particularly important for emerging artists seeking recognition in a saturated market.
The intelligent recommendations provided by music finders can lead to serendipitous discoveries, thereby broadening the musical horizons of the listener.
Moreover, these platforms leverage data-driven insights to assist marketing strategies for record labels. As the demand for personalization grows, the data curated by similar music finders become instrumental in shaping trends. Artists gathered user feedback through these platforms can adapt their offerings, reaching their target audience more effectively. In summary, understanding similar music finders is crucial for anyone looking to navigate today's diverse musical environment.
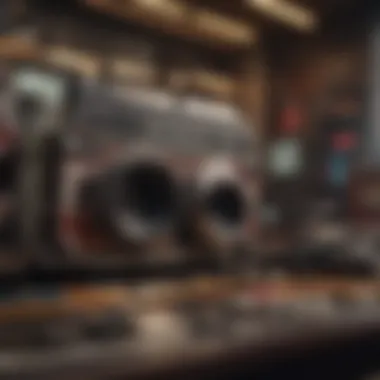
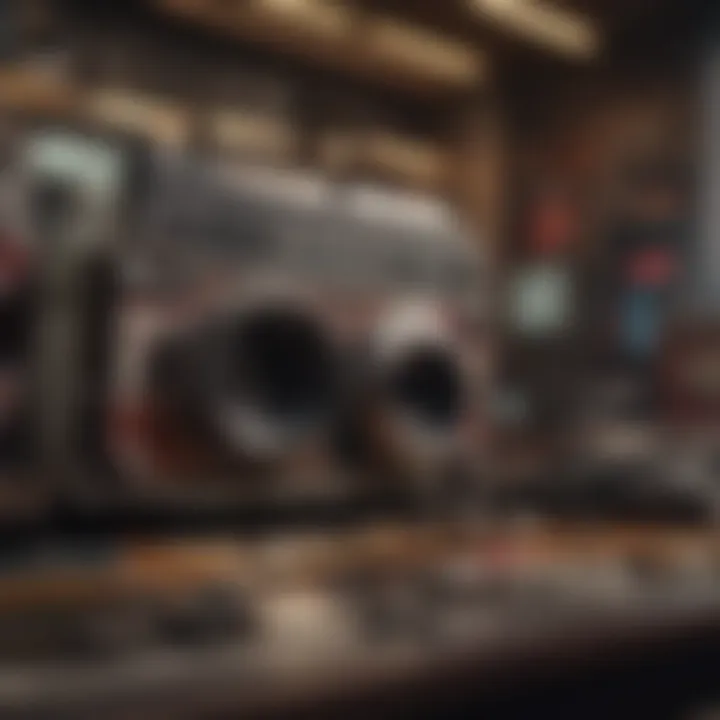
How Similar Music Finders Operate
Understanding how similar music finders operate provides valuable insights into their functionality and effectiveness. These platforms employ advanced methodologies to analyze and recommend music tailored to individual user preferences. Gaining knowledge in this area not only informs users about the technology behind music recommendations but also enhances their experience in discovering new tracks and artists that align with their sounds.
Algorithmic Foundations
Algorithmic foundations represent the core structure powering music finders. These algorithms use various mathematical models and techniques to process user data and deliver personalized suggestions. Here are key elements to consider regarding algorithms in music finders:
- Recommendation Algorithms: Many platforms utilize collaborative filtering and content-based filtering. The former mirrors preferences of similar users, while the latter focuses on the characteristics of the music itself.
- Machine Learning: Innovations in machine learning allow algorithms to learn from user interactions over time. These systems evolve and improve recommendations based on accumulated data points like likes, skips, and repeated plays.
- Meta-Data Analysis: Tik uses meta-data, such as song genres, release dates, BPM, and artist collaborations, to draw connections and suggest tracks that resonate with listeners. This depth of analysis creates a more informed base for music recommendations.
Understanding how algorithms function empowers users. It helps them navigate platforms more effectively and shape musical exploration towards personal tastes.
Data Sources and User Input
The functionality of similar music finders largely hinges on diverse data sources and user input. These elements interweave to form an interdependent relationship that drives precise music suggestions.
First, data sources may include:
- Streaming Metrics: Platforms accumulate vast amounts of streaming data that records user behavior, identifying trending tracks and artists.
- Social Interaction: User interactions on platforms, especially likes, shares, and comments, contribute significantly to determining music trends and tastes.
- Contextual Information: Some systems, like Spotify’s Discover Weekly, adapt to specific user contexts, such as time of day or listening moods, forming a more holistic view of their preferences.
Next, user input plays a crucial role. By deliberately curating their listening habits or providing direct feedback, users influence the algorithms to tailor results:
- Creating Playlists: Users can create playlists of favorite tracks, providing explicit data that algorithms can leverage for better suggestions.
- Rating and Feedback: Many platforms allow users to rate songs or provide feedback, thus refining future recommendations.
- Exploring Genres: By traveling through various genres, listeners signal their musical desires, prompting the finder to identify similar tracks in those areas.
By merging diverse data inputs with analytical users’ actions, similar music finders can deliver something close to a personalized auditory experience, enriching the journey of discovering new artists and music styles.
Benefits of Using Similar Music Finders
Using similar music finders provides various advantages that fundamentally improve how listeners engage with music. In a world overflowing with tunes and artists, navigating the musical landscape can feel overwhelming. These tools simplify the discovery process, allowing users to find what resonates with them personally. By understanding the benefits of these tools, individuals can tailor their listening experiences based on preferences and interests, enriching their auditory exploration.
Enhanced Music Discovery
Similar music finders contribute significantly to enhanced music discovery. These systems aggregate songs and artists by analyzing distinct elements, including sound patterns, common features, and lyrical content. The discovery aspect allows users to explore genres they may not initially consider. For instance, a user who enjoys indie pop may discover alternative rock bands that share similar arrangements or instrumentation. This exposure enriches the listening process, bringing fresh perspectives and sounds, ultimately reducing the risk of musical stagnation.
Content curation not only introduces users to new artists but also creates a ripple effect of further explorations. As listeners engage with these recommendations, they become part of a self-reinforcing cycle of music discovery. Engaging with peers, platforms like Last.fm or Reddit can be useful. By sharing their experiences and discoveries, users contribute to a richer learning environment.
Personalized Listening Experience
A hallmark of similar music finders is their ability to provide a personalized listening experience. Each platform leverages individual user data to curate suggestions that align closely with their tastes. For example, Spotify Discover Weekly utilizes algorithms to offer a personalized playlist every week based on past listening habits. This attention to personal preferences makes users feel understood, fostering a deeper connection to the music they listen to.
The rise of user-tailored recommendations means that every individual can support their unique style in music. This concentration keeps boredom and repetition at bay while providing dynamic content. As users continue interacting with platforms, the personalization grows sharper, evolving over time to reflect changes in preferences or emerging trends in a user’s life. Keeping updates and modifications in mind is essential. Users learn from each interaction to question what types of songs/musical styles engage them the most, allowing for deeper listening joy.
Expanding Musical Horizons
Through similar music finders, listeners gain access to a vast array of genres and styles previously outside their known preferences. The importance of expanding musical horizons cannot be overlooked. Through variance in what users are offered, the constraints that once restricted their exploration begin to dissolve.
The integration of world music, experimental genres, and underground scenes fosters an environment ripe for experimentation. Knowledge gained through these platforms empowers listeners to embrace new flavors and continue expanding their mental Libraries of sound. This open-mindedness can be profoundly gratifying and intellectually enriching.
Listening identifies commonalities amongst genres and reveals influences lurking in unexpected sounds. Whether one chooses to explore deeper scenes or catch main music trends, using these finders can illuminate paths unable to be traversed otherwise, ensuring that every listening adventure sparks reflection and growth.
When used mindfully, similar music finders turn routine listening habits into a thrilling exploration of new musical landscapes. Fueling outcomes based on analysis can lead long-term to impressionable musical favorites.
Challenges Faced by Similar Music Finders
Similar music finders occupy a crucial space in the contemporary digital music ecosystem. While they provide a promising approach to uncover new tracks, they also face a range of challenges that can limit their effectiveness. Understanding these challenges is vital for users and developers alike. Addressing issues related to music algorithms, user needs, and recommendation quality enhances the appreciation and functionality of these tools. Striking a balance helps ensure that the exploration experience remains enriching and tailored.
Over-Reliance on Algorithms
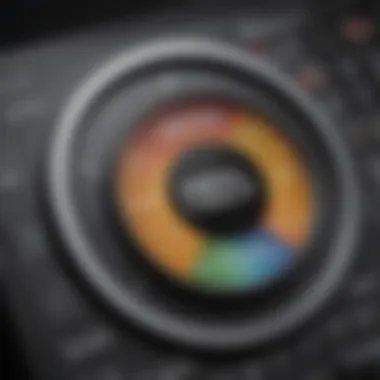
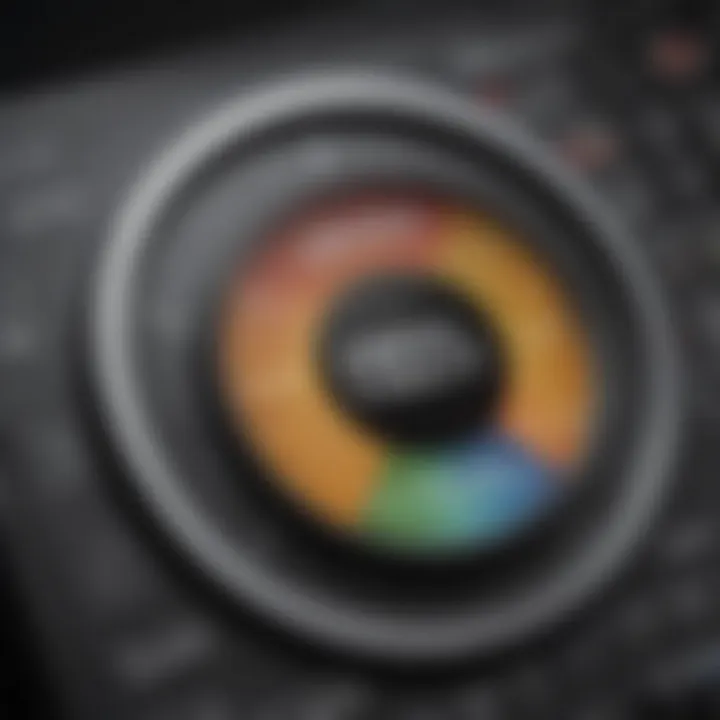
Algorithms form the backbone of many similar music finders today. They analyze vast amounts of data to identify patterns and suggest music options. However, an over-reliance on algorithms poses various risks. For instance, algorithms can sometimes lead to homogenized listening experiences. If users only receive recommendations based on past behavior, there's a chance they miss out on eclectic and diverse genres. This situation can result in music echo chambers, stunting growth and broaden in musical tastes.
Moreover, algorithms may not capture the nuanced emotions of specific songs. Different people experience music uniquely due to cultural backgrounds, personal experiences, or even mood at a given time. Algorithms tend to quantify preferences, but they often fail to appreciate this vast complexity, potentially leading to recommendations that lack authenticity or relevance. Finding an effective balance between algorithmic design and a more personalized touch is crucial.
Understanding User Preferences
Gaining insight into user preferences is essential for any successful music recommendation system. User preferences extend far beyond just liking a certain genre. They involve a spectrum of factors, like emotional states and social contexts. Failing to adequately understand what drives user engagement compromises the quality of recommendations. To improve this understanding, users should actively participate in providing feedback on their listening habits and choices.
Moreover, platforms that emphasize user input are likely to see a higher satisfaction rate in their recommendations. Listener habits might evolve, and so too should the approaches of these music finders. Regular updates based on user feedback ensure that the playlists and suggestions remain relevant and dynamic. Music is inherently subjective, and a truly effective platform takes this into account.
Quality of Recommendations
Maintaining a high level of quality in music recommendations is another prominent challenge faced by similar music finders. There is a tendency toward popularity bias, where the system tends to recommend only the most well-known tracks or artists. This situation disserves users looking for fresh voices or lesser-known talent.
A careful calibration of recommendations is essential. This cannot solely rely on algorithms but must also incorporate human editorial insight. Additionally, integrating music industry expertise can facilitate curated recommendations that benefit both listeners and emerging artists alike. Encouraging diversity in exposure not only enriches user experiences but also promotes a vibrant musical landscape.
With a critical understanding of these challenges, users can better engage with similar music finders. Recognizing the limitations empowers users to mix and match their sources for a fuller musical experience.
Current Popular Similar Music Finders
Finding new music through tools that suggest tracks similar to those you already love is central in today’s digital sound landscape. Knowing what options exist allows users to either explore their favorite genres more deeply or discover entirely new ones. Each platform employs individual approaches to curate and suggest tracks, resulting in unique user experiences. Below are some prominent players in the similar music finder space.
Spotify Discover Weekly
One of the most recognized features within the Spotify ecosystem is Discover Weekly. It creates personalized playlists for users, refreshed every Monday. The algorithm analyzes listening habits alongside user profiles and adapts over time.
- Key Features:
- Bi-weekly updates of playlists
- Algorithmic curation based on listening history
- Seamless integration with other Spotify features
This service effectively combines human-like recommendations with data-driven selection, which leads to a rich exploration of music tailored for each user.
Pandora
Pandora was one of the early innovators in streaming music discovery. Users can create personalized radio stations by selecting a favorite track or artist. The platform employs the Music Genome Project, which analyzes songs across over 400 attributes.
- Key Features:
- Create radio stations from songs, artists, or genres
- Human-curated playlists available alongside algorithmic suggestions
- Ability to thumb-up or thumb-down to fine-tune preferences
Pandora provides a solid blend of automatized recommendations and human touch by using complex music characteristics to deliver satisfying listening experiences.
Last.
fm
Last.fm serves as a large music community resource, tracking users' listening habits through scrobbling — a feature that registers what songs you play from various platforms. It utilizes this data to suggest songs, artists, and events.
- Key Features:
- User-generated data focuses on listening patterns
- Extensive artist information and similar recommendations
- Connection with other users for discovery via shared tastes
This platform enables a highly personalized experience based on collaborative filtering, further enriching music discovery.
SoundCloud Explore
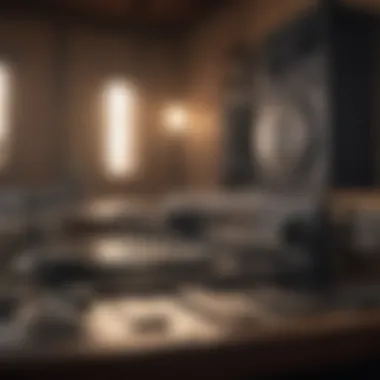
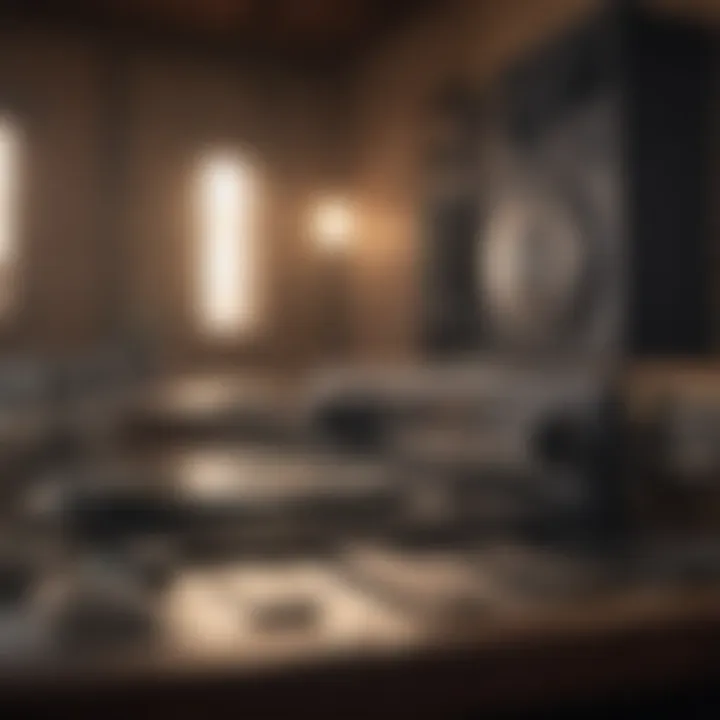
SoundCloud stands out due to its performance as an emerging artist platform host. Beyond personal playlists, its Explore feature offers recommendations aligned with trends and community interactions.
- Key Features:
- Trending music and emerging artist focus
- Curated playlists and community-based activity
- Easy sharing capabilities within user network
Through organizing tracks from lesser-known creators alongside mainstream ones, SoundCloud provides unique opportunities to uncover raw and original sounds.
Understanding these platforms positions users to maximize their experience, creating opportunities for deeper music connection and variety.
Maximizing the Use of Similar Music Finders
Maximizing the use of similar music finders is pivotal in enhancing the music discovery experience. These tools offer vast potential for broadened listening choices. However, to fully benefit from such features can require a strategic approach. This is where maximizing their use comes into play, influencing listeners' interaction with music discovery platforms, and enriching their overall musical landscape.
Setting Proper Expectations
When using similar music finders, users should establish clear expectations. These tools are designed to suggest music that aligns with user preferences. However, it is vital to recognize that not all recommendations will resonate. By accepting this reality, users can appreciate the serendipity involved. Moreover, discovering music often entails exploring outside one's typical genres. Setting intentions around openness will enable further rich discoveries. Always keep in mind that a song that initially seems unfamiliar might lead to hidden gems.
Mixing Algorithms with Human Curation
The best results come when algorithms and human curation blend together. Relying solely on algorithms can lead to repetitive suggestions, limiting new music exploration. Instead, engaging with platforms that implement human oversight can offer a refreshing perspective on recommendations. Playlist creators often understand nuances that machines might overlook. A collaborative approach encourages users to
The Future of Music Discovery
Music discovery is at a pivotal point in its evolution. With the rapid advancements in technology, the methods we employ to unearth new soundscapes are changing. The future of music discovery promises to bring both challenges and opportunities. The potential for better personalization, enhanced recommendations, and novel ways to connect with music is vast. For listeners and artists alike, understanding these trends is vital.
Emerging Technologies in Music Finding
The music industry is increasingly incorporating emerging technologies to improve the way music is found. Tools powered by artificial intelligence and natural language processing are creating a more intuitive experience for users. These technologies aid in parsing user data, understanding musical preferences, and generating personalized playlists.
Recent advancements allow platforms to draw insights not just from listening habits, but also from social media and other digital footprints. This multifaceted approach broadens the scope of recommendations beyond mere genre classifications.
Experts predict that the adoption of virtual reality (VR) and augmented reality (AR) will provide even more immersive ways to experience music. Users might soon enjoy virtual concerts or music-related environments tailored to their tastes. There is also growing investment in practical applications of machine learning algorithms, known for recognizing patterns and predicted outcomes, to make music discovery more effective.
Integration of Machine Learning
Machine learning algorithms serve as the backbone of many music discovery platforms. By analyzing large datasets, these algorithms learn from users’ listening behavior, identifying trends and preferences that inform suggestions.
The feedback loop is crucial here. When users interact with the recommendations–either by liking, disliking, skipping, or saving songs–these actions are fed back into the system. This data allows for the refinement of future recommendations, making them increasingly relevant and nuanced.
This ongoing learning cycle results in a tailored experience that adapts to users' auditory tastes over time. Consequently, platforms become more effective at introducing fresh sounds without consuming valuable time or effort searching for new music. In a landscape where attention spans are shortening, such efficiencies become essential for user retention and satisfaction.
Implications for Listeners and Artists
The trends in music discovery are poised to affect both listeners and artists significantly. For listeners, hyper-personalization can lead to deeper connections with music. The likelihood of discovering lesser-known artists increases, giving people more access to diverse musical expressions.
On the side of artists, enhanced discoverability could change the music landscape. Artists may find themselves supported by platforms that elevate new talents, making it easier for them to reach audiences beyond geographical boundaries.
Moreover, analytics provided by these platforms can help artists understand their listeners better, possibly informing their future work, marketing strategies, or tour decisions. In essence, the synergistic relationship between listeners and artists will likely strengthen as technology evolves.
The End
The exploration of similar music finders offers significant insights into the evolving dynamics of how we discover and interact with music. The discussed aspects underpinning this topic illustrate both the technological advancements and the human penchant for musical exploration. Understanding these nuances can empower listeners to navigate their tailored music journey effectively.
Summary of Key Insights
In synthesizing the article's content, several core insights emerge:
- Functionality: Similar music finders leverage algorithms and vast data to suggest music tailored to individual tastes.
- Enhanced Experience: These platforms aim to enhance music discovery, offering personalized recommendations that expand users' listening horizons.
- Challenges: However, there are challenges to consider, such as maintaining quality in recommendations and avoiding over-reliance on technology alone.
- The Future: Advancements in machine learning will further enrich these tools, potentially forging an even deeper connection between listeners and the music that resonates with them.
The Ongoing Journey of Music Discovery
Music discovery is not a static endeavor; it is an ongoing journey influenced by changing tastes, technological innovations, and individual experiences. Each platform introduced continuously adapts and provides opportunities to explore unfamiliar sounds. As listeners engage with these tools, their preferences evolve, fostering a relationship built on curiosity and appreciation.
This journey is unique for every individual. Engaging with various music finders can uniquely shape how one perceives and enjoys the art of music.